Introduction
When thinking about machine learning, you would be forgiven for thinking that it’s something used exclusively for large and complex projects like, for instance, NASA’s path planning of robots deployed to other planets. You might even think of it as something out of a science-fiction film where machines have taken over the world. In reality, machine learning is everywhere and you are reaping the benefits in your daily life.
Google, a major player in machine learning, are trying to build it into all of their products. Their applications range from improving search engine functionality to powering self-driving cars. In a series of recent AI acquisitions, Google bought London-based artificial intelligence firm Deepmind technologies, whose AlphaGo program defeated the strongest Go player in the world yet again this year. This was a huge milestone in machine learning as Go is a notoriously difficult game for computers to play, largely due to there being more legal board positions in the game than atoms in the universe.
Amazon uses machine learning algorithms to suggest products you might want to buy based on your purchase history and what it’s learnt from all of the previous shoppers before you. Netflix and Spotify also use a similar approach to build personalised playlists. Twitter can tell businesses what their customers are saying about them. Machine learning is also used in ecommerce for fraud detection, whilst hedge funds are using it to predict moves in the stock market.
But what exactly is machine learning? In essence, it’s a set of algorithms that machines can use to learn and make predictions on data without being explicitly programmed to do so. It means machines can learn to perform complex tasks such as face recognition and text translation, which are very difficult to program conventionally.
Machine learning is not something new and the theory for it has been around since the 1950s. The trouble at the time was having hardware powerful enough to put any of it into practice. Its recent explosion is a result of growing volumes of data and computational power being cheaper and more powerful than ever. This means we can analyse more data and produce better models, resulting in more accurate predictions.
Machine learning in education
Machine learning in education is not a new concept. The fields of Learning Analytics (LA) and Educational Data Mining (EDM) go back to the start of the millennium but, like everything machine learning related, they have been growing in popularity lately. Dr Ryan Baker of Columbia University launched the course “Big data in education” on Coursera in 2013 (recently moved to edX) which goes into how to apply these fields to large-scale educational data.
Pardos et al. investigated how affective states of middle school students (11-13 year olds) can predict end of year learning outcomes. Log files from the ASSISTments maths tutoring system were analysed to develop behavioural detectors for affective states such as boredom, frustration, confusion, and engaged concentration. The behavioural data was then correlated with end of year exam results in order to examine its impact on performance. Unsurprisingly, the data showed that students who were bored or confused while solving problems did poorly in their exams. However, exhibiting the same behaviours while receiving support was associated with positive performance. This highlights the importance of context when examining such behaviours. The study suggests that machine learning could be used in real-time to analyse such data and feed indicators in teacher dashboards, enabling them to make early interventions.
Machine learning in an LMS
Why would we want to use machine learning in an LMS? Well, we’re sitting on a gold mine of data about our learners and their use of our platforms. At the moment, apart from creating fancy looking dashboards, we are only scratching the surface of what we can use it for. Machine learning can help us unlock these insights and allow us to better understand the use of our learning materials and predict how our learners might behave.
Similarly to the Pardos study, behavioural data in a corporate LMS could be classified and correlated with an employee’s performance review. This can tell us what behaviours are associated with poor performance and raise early warning signs for intervention.
Course recommendations are not a novel idea and already appear in many modern LMS platforms. These recommendations can be made more relevant by using Netflix/Spotify style machine learning algorithms and studying users’ behaviour. The early intervention idea can be taken further here by recommending courses to users in order to improve performance.
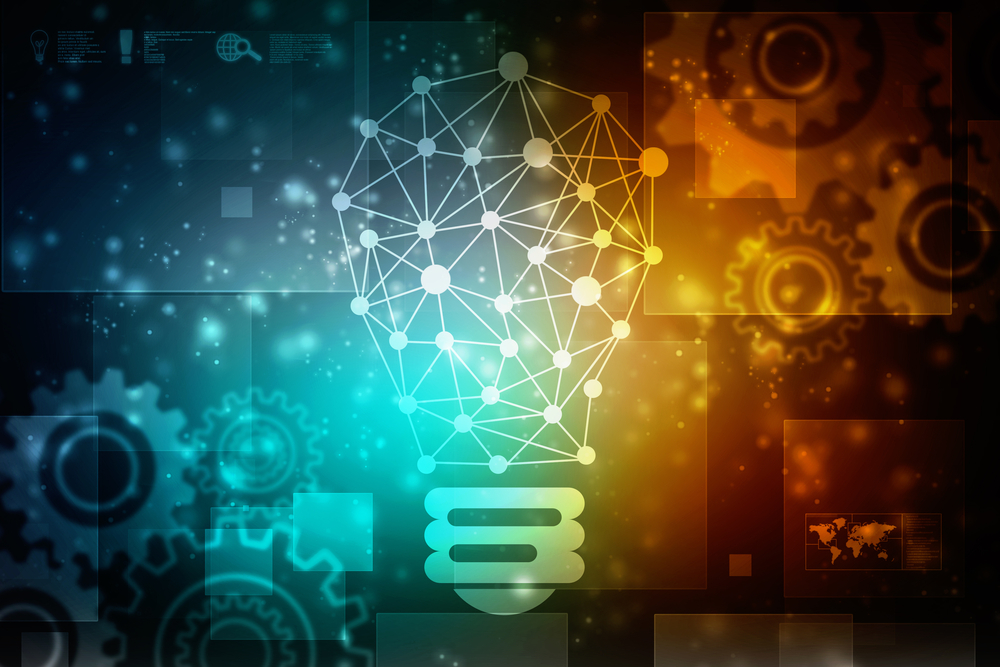
Social learning
I’m sure you’ve already heard why social learning is so important for business and even if you’re not doing anything about it, it’s still happening daily in your organisation in the form of informal face to face knowledge sharing. The problem with informal training like this is that nothing is logged and we are missing a huge opportunity to find out more about our learners.
To help us see how this data can be utilised, we can take a look at how popular social networking websites are already using machine learning in their products. Facebook have created the FBLearner FLow platform and are using it for tasks like content moderation, sorting of content by relevance and grouping similar content – these can all be used to improve user engagement. Twitter data is being used for sentiment analysis, which can help tell what your customers are saying about you. Within an organisation, sentiment analysis can be used to tell what your employees think about recent changes, their satisfaction with the level of support provided, or even what they think about the available training.
The topic of user engagement is crucial when looking at introducing machine learning to your LMS. Not only is it an important factor in establishing a return on investment for any platform, but a lack of engagement will result in significantly less data being available for analysis and inhibit the accuracy of predictive models. Techniques such as personalisation and gamification have proved effective in keeping people coming back but, more importantly, regular fresh content is essential in maintaining their interest. This is where the social functionality can again play a big role as it allows for user generated content to fill the gap between official training being added to the platform. User playlists can give learners a chance to become the teacher by collating resources and sharing their knowledge with peers.
The future
With computational power and data storage becoming increasingly cheaper and more abundant, and the world’s hottest tech firms investing so heavily in machine learning, what does the future hold? Deep learning, a type of machine learning primarily inspired by biological neural networks such as the brain, promises to lead the way. It requires very large datasets and is typically used for complex tasks such as face recognition, speech recognition, and scene parsing. In the future, it could be used for tasks like real-time speech translation which could eradicate language barriers.
We’ve looked at how machine learning can have an impact on LMS right now, but what could further advancements in the field hold? Personal training assistants like Siri or Cortana could become the norm, being able to guide learners through their training and provide support when required. Richer data logging will be required for more accurate behaviour detection and more interesting predictions. To aid in this, we will see a rise in the use of central learning repositories such as Learning Record Stores (LRS) for the Experience API (TinCan). These allow for a much more comprehensive collection of data about learners, both formal and informal. Being signed into something like this on a phone, would allow learning to be tracked on the go and even offline. What is really significant here is that the LRS can persist between systems and devices and build up data about a learner over their entire lifetime.
Over the past decade we have seen a surge in factory workers being replaced by robots. Repetitive mechanical tasks can be carried out more efficiently by robotic arms on automated production lines – they never tire and rarely make mistakes. Apple and Samsung supplier Foxconn has just replaced 60,000 factory workers with robots and is now becoming an ever increasing trend in China. The rise of machine learning will take this further with a 2013 report suggesting that 47% of US jobs are at risk of automation. Any repetitive tasks now carried out by humans can be learnt and automated. eLearning and online courses have already contributed to the teacher role diminishing to that of a facilitator – will an organisation still require L&D professionals when an out of the box LMS is capable of identifying and providing all of the necessary training?
With machine learning coming into the mainstream fast, are you ready to take full advantage? SoftServe, a software and application development specialist, reports that 62 percent of medium to large organisations that it surveyed are expecting to roll out machine learning tools for business analytics by 2018. It’s important not to let learning technologies in your organisation fall behind this trend. Contact us to find out how Saffron’s digital solutions can keep you at the cutting edge.
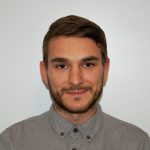
Pavlo Onyshchenko
Developer and emergent technology researcher
Pavlo is an experienced back-end and front-end developer in .net, PHP, JavaScript and HTML5 who has built customised database architecture solutions, new plugin sets and system integrations. Noted for his attention to detail and project management skills, he was instrumental in the development of a bespoke LMS for Aldi. Pavlo is always casting a keen developer’s eye on the latest digital technologies, appraising their value for a learning environment. A keen climber, he employs that endurance and eye for the optimal route as he successfully summits project after project.